Mission
Our objective in this group is to leverage the data and the knowledge emerging from “human sensors” while also tackling the innate opportunities and tough problems they generate; like for example diversity and privacy. It is estimated that by 2020, by interacting with the ever more connected and data driven world where we live, each singular individual will generate 1.7 megabytes of data per second . Humans themselves; through their online presence, use of devices, and social interactions; are indeed becoming the ultimate “sensors“ for capturing data about the world.
Research Directions
Currently, the activities of the K-LAB can be grouped according to the following research directions:
- Data and Knowledge acquisition, representation and discovery. This area is mainly concerned with the optimal strategies for capturing the massive amount of data being generated, then representing it and storing it in formats that are maintainable and that facilitate their future analysis. All of this towards learning and discovering higher-level data and knowledge (like context and social relations) that can be fed again into the capture/learning loop. As explained in more detail in the papers and projects below, to do so we use techniques from Knowledge Representation, Big Data analysis, Machine Learning, among others.
- Personal data and privacy management. A lot of the data generated by humans, has personal information intrinsically connected to it. Unlike regular data Personal data has the additional problem of its use being regulated by various, and sometimes contradictory, laws around the world. The most common approaches to manage Personal data involve reducing or removing this personal component (i.e., pseudonymization and anonymization). Nevertheless, removing/reducing personal information also impacts the transparency and possible utility that such data had. In this research line we are interested in managing Personal data in accordance with applicable privacy protection regulations while still maximizing its transparency and utility.
- Experiment execution and management. The human component is an often ignored in computer science. This research direction, in collaboration with the department of sociology of the university of Trento, is focused on understanding the best practices and methodologies involved in running experiments/pilots with human participants.
Main Publications
- Mattia Zeni, Ivano Bison, Britta Gauckler, Fernando Reis, and Fausto Giunchiglia. "Improving time use measurement with personal big data collection - the experience of the European Big Data Hackathon 2019." Journal of Official Statistics, 2020.
- Zeni M, Zhang W, Bignotti E, et al. Fixing mislabeling by human annotators leveraging conflict resolution and prior knowledge[J]. Proceedings of the ACM on Interactive, Mobile, Wearable and Ubiquitous Technologies, 2019, 3(1): 1-23.
- Giunchiglia, F; Zeni, Mattia; Gobbi, Elisa; Bignotti, Enrico; Bison, Ivano. Mobile social media usage and academic performance. Computers in Human Behavior, vol. 82, p. 177-185, 2018.
- Giunchiglia F, Zeni M, Big E. Personal context recognition via reliable human-machine collaboration[C]//2018 IEEE International Conference on Pervasive Computing and Communications Workshops (PerCom Workshops). IEEE, 2018: 379-384.
- Giunchiglia F, Bignotti E, Zeni M. Personal context modelling and annotation 2017 IEEE International Conference on Pervasive Computing and Communications Workshops (PerCom Workshops). IEEE, 2017: 117-122.
- Zeni M, Zaihrayeu I, Giunchiglia F. Multi-device activity logging Proceedings of the 2014 ACM International Joint Conference on Pervasive and Ubiquitous Computing: Adjunct Publication. 2014: 299-302.
- Kim P H, Giunchiglia F. The open platform for personal lifelogging: the elifelog architecture[M]//CHI'13 Extended Abstracts on Human Factors in Computing Systems. 2013: 1677-1682.
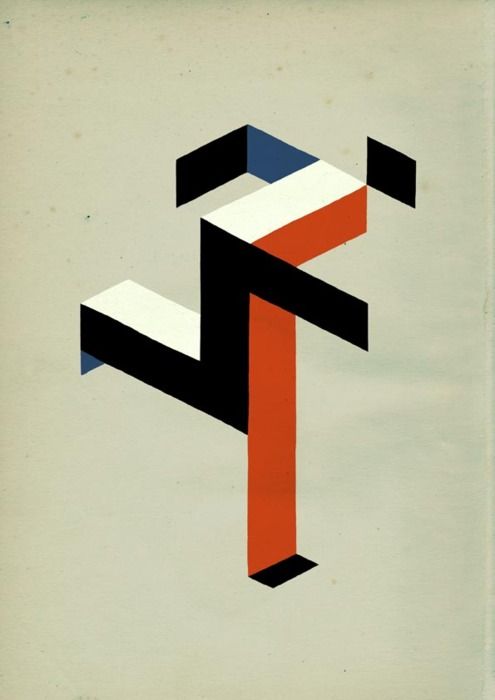
Lead by
Members
Topics
- Knowledge Representation and Reasoning
- Stream data management and optimization
- Machine Learning
- Applied Ontology
- Cognitive Modeling
- Experiment management
Related Projects