Xiaoyue Li – Trentino spatial datasets introduction and tentative integration process (54:56)
07/06/2023
This seminar is to introduce the work about how to generate comprehensive Trentino location datasets. We start from the OpenStreetMap resource and design the general Light Weight Ontology (LWOntology), which is the general knowledge graph organizing and representing datasets from a semantical level. The real datasets are separated based on the general LWOntology and then mapped with it. We use Trentino OpenStreetMap datasets as an example to implement the general LWOntology, where we generate Trentino LWOntology, and map Trentino OpenStreetMap datasets with Trentino LWOntology. Furthermore, the general LWOntology will provide the methodology to integrate location datasets from different resources, e.g., Trentino OSM datasets can be composed with Trentino POI datasets and Trentino GTFS datasets.
Slides
Andrea Bontempelli – Concept-level Debugging of Part-Prototype Networks (57:19)
29/03/2023
Part-prototype Networks (ProtoPNets) are concept-based classifiers designed to achieve the same performance as black-box models without compromising transparency. ProtoPNets compute predictions based on similarity to class-specific part-prototypes learned to recognize parts of training examples, making it easy to faithfully determine what examples are responsible for any target prediction and why. However, like other models, they are prone to picking up confounders and shortcuts from the data, thus suffering from compromised prediction accuracy and limited generalization. We propose ProtoPDebug, an effective concept-level debugger for ProtoPNets in which a human supervisor, guided by the model’s explanations, supplies feedback in the form of what part-prototypes must be forgotten or kept, and the model is fine-tuned to align with this supervision. Our experimental evaluation shows that ProtoPDebug outperforms state-of-the-art debuggers for a fraction of the annotation cost. An online experiment with laypeople confirms the simplicity of the feedback requested to the users and the effectiveness of the collected feedback for learning confounder-free part-prototypes. ProtoPDebug is a promising tool for trustworthy interactive learning in critical applications, as suggested by a preliminary evaluation on a medical decision making task.
Ivan Kayongo – Supporting Everyday Life Awareness (28:13)
22/03/2023
Integrating personal sensor data with digital calendars and visualization can improve the extraction of insights and comprehension of personal behavior. Different people have different needs and aligning their plans with the actual execution can help them better understand their lifestyles. Digital calendars provide a more effective display of an individual’s behavior patterns and allow for easy navigation of the data along their life timeline and understand their life.
Matteo Busso – Generating and sharing person centric data (47:47)
13/03/2023
DataScientia Project – Seminar – LivePeople research infrastructure for South Africa.
Xiaoyue Li-Episode-based personal data streams formalization (35:05)
23/11/2022
Smart devices, e.g., smartphones and smartwatches, provide large-scale personal data streams, including (objective) sensors from machines and (subjective) annotations from people. This seminar reports our first step work for organizing, reasoning, and learning from personal data streams to represent people’s daily life. We first introduce one of our proposed personal situational theories: episodic, which is the minimal KG model to represent a scenario. Then this seminar will show how to use the data resource (SmartUnitn2 dataset) and the knowledge resource (episode model) to generate an artificial dataset representing users’ daily lives. The generated dataset starts from the SmartUnitn2 dataset and with the features of episode theory.
Slides
More videos on this topic
Xiaoyue Li-A Context Model for Personal Data Streams (09:31)
The Asia Pacific Web (APWeb) and Web-Age Information Management (WAIM) Joint International Conference on Web and Big Data (APWeb-WAIM)
27/11/2022 Venue:Nanjing China
We propose a model of the situational context of a person and show how it can be used to organize and, consequently, reason about massive streams of sensor data and annotations, as they can be collected from mobile devices, e.g. smartphones, smartwatches or fitness trackers.
About APWeb-WAIM
Slides
More videos on this topic
Matteo Busso – iLog as a service – Service Perspective (47:43)
26/10/2022
Current technologies have the potential to make people aware of their everyday life, with enormous benefit in many activities and in decision making process. Indeed, technologies can support awareness through developing models, tools, devices, and applications to have better management of people context information. A current approach to enhance the technologies is to involve people in processing their data (human-in-the-loop), so that people understand better their own collected information and have ways to verify and analyse them.
However, current personal data management methodologies assume expert knowledge of the topic, which is often composed of complex notions and tasks. This is one of the reasons why people are often involved as contributors of information and not as actual creator.
We aim to build a process and a set of easy to understand and easy to use tools, so that people can be active and informed participants in the data and information management processes. Our contribution aims to give the technological tools and skills to anyone who wants to use them to increase people’s knowledge and awareness.
Slides
More videos on this topic
Marcelo Rodas – iLog as a service – Platform Perspective (59:01)
19/10/2022
Everyday life awareness has always been the main concern for humans. Current technologies join this concern by developing models, tools, devices, and applications to have better management of people context information. A current approach to enhance the technologies is to involve people in processing their data (human-in-the-loop), so that people understand better their own collected information and have ways to verify and analyze them. iLog System was conceptualized as a tool to represent the user context information using technological devices and questionnaires. With this tool we want to provide services for Researchers to follow the full cycle of any user behavior study: experiment design, data collection, data preparation, and data analysis. The overall goal is to build a painless symbiosis between the rigorous and systematic scientific process and the human concern for their everyday life awareness using the best technological tools.
Slides
More videos on this topic
Andrea Bontempelli – Interactive Label Cleaning with Example-based Explanations
Machine Learning Group Journal club (56:57)
10/06/2022
Structured Machine Learning Group Journal club.
|
Slides
More videos on this topic
Xiaoyue Li – Representing Habits as Streams of Situational Contexts
Caise workshop-ISESL (19:02)
06/06/2022
Caise workshop-ISESL.
|
Slides
More videos on this topic
Ivano Bison – Citizen Science: a platform for accessing sensors from mobile devices
the fourteenth edition of the National Statistical Conference (1:26:31)
01/12/2021
About the National Statistical Conference
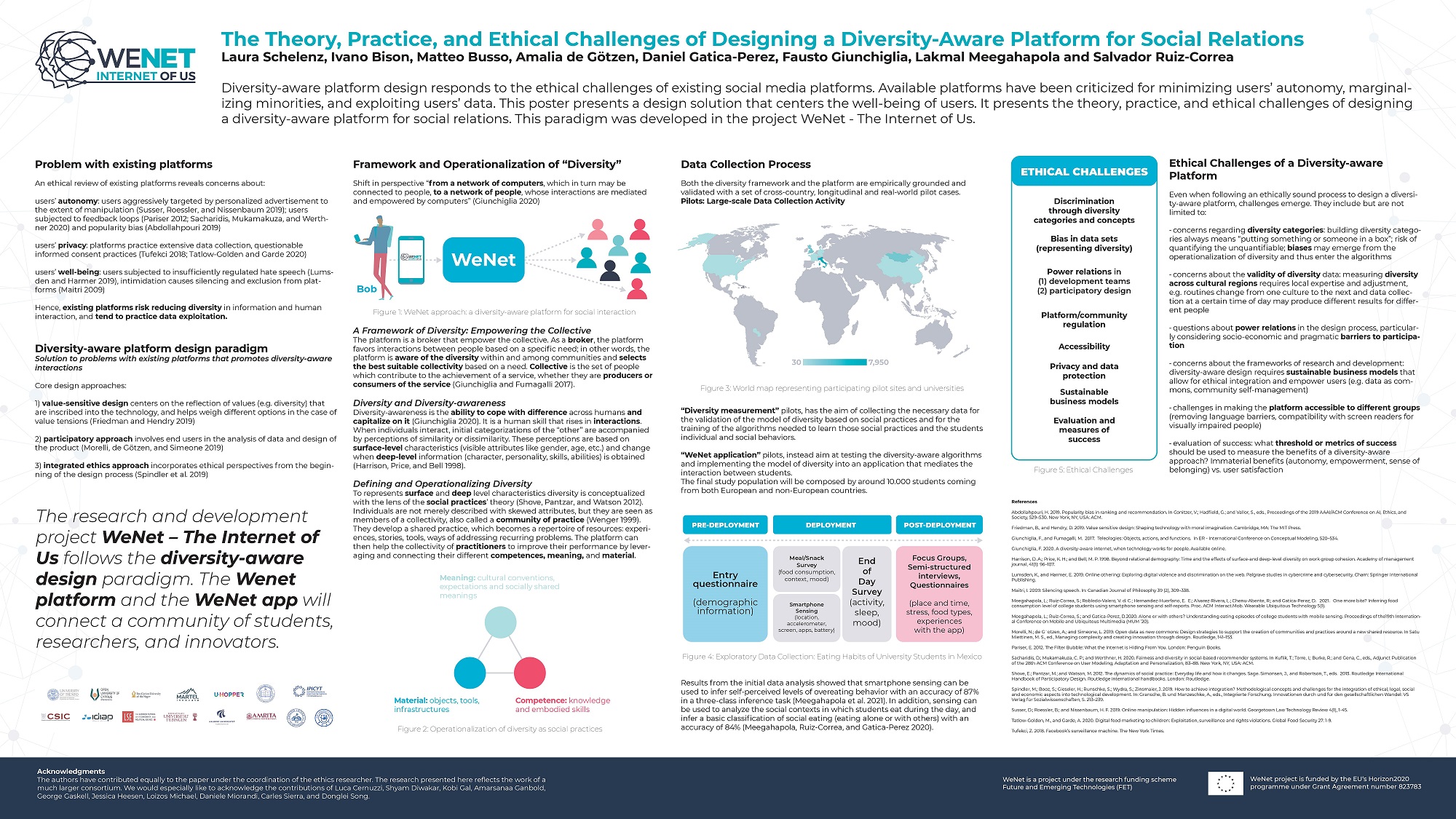
Matteo Busso – The Theory, Practice, and Ethical Challenges of Designing a Diversity-Aware Platform for Social Relations
AIES 2021 – Proceedings of the 2021 AAAI/ACM Conference on AI, Ethics, and Society – POSTER SESSION
19/05/2021
AAAI/ACM Conference on AI, Ethics, and Society
More videos on this topic
Andrea Bontempelli – Learning in the Wild with Incremental Skeptical Gaussian Processes
IJCAI-2020 (14:10)
07/01/2021
This paper addresses the task of incremental classification in the wild by a redesign of skeptical learning centered around Gaussian Processes (GPs).
Find the paper
|
pdf
About the IJCAI-2020